Breaking the Monopoly: Open-Source AI’s Challenge to OpenAI’s Dominion

The emergence of open-source large language models (LLMs) is redefining the AI landscape. Gone are the days when proprietary models like OpenAI’s ChatGPT held the monopoly in AI-driven solutions. Today, a paradigm shift is underway as businesses increasingly turn towards open-source LLMs for their versatility, customization capabilities, and cost-effectiveness.
This transition is not just a fleeting trend but a significant movement towards democratized AI. Open-source models offer a unique blend of transparency, adaptability, and community-driven development, which stands in stark contrast to the ‘black-box’ nature of closed models. While the latter have been lauded for their sophistication and ease of use, the former are gaining ground for empowering businesses with the freedom to modify and tailor AI models to their specific needs.
The journey towards embracing open-source LLMs hasn’t been without its challenges. Initial hesitancy, concerns about support and maintenance, and the need for specialized expertise have been significant hurdles. However, as the technology matures and success stories begin to surface, the business world is increasingly recognizing the potential of open-source LLMs.
The Slow Adoption of Open Source Language Models
The journey towards integrating open-source Large Language Models (LLMs) in business operations has been a path of gradual discovery and cautious advancement. This slow adoption can be attributed to a variety of factors, which, upon closer examination, reveal a complex tapestry of challenges and opportunities.
Initial Hesitance and Challenges
Initially, enterprises exhibited a noticeable hesitance toward open-source LLMs. This reluctance primarily stemmed from concerns over support and maintenance. Unlike their closed-source counterparts, open-source models often lack the dedicated support and service guarantees that come with commercial products. Businesses, especially those without robust in-house technical teams, found this aspect daunting.
Another significant hurdle was the maturity of the technology. The first major open-source model from Meta, Llama, was released in February 2023, which lagged behind the release of OpenAI’s ChatGPT. This delay meant that open-source LLMs were initially playing catch-up, both in terms of technological development and market presence.
Integration and Compatibility Concerns
Integrating open-source LLMs into existing business systems presented another challenge. Companies often found that aligning these models with their specific workflows and data ecosystems required significant customization. This need for tailoring, while offering flexibility, also introduced complexity and potential resource strain.
Moreover, the open-source nature of these models raised questions about compatibility and ongoing development. Businesses wondered how these models would evolve and whether they would be able to keep pace with rapidly changing market needs and technological advancements.
Perception of Risk
Risk perception played a substantial role in the slow adoption. Open-source models, by their very nature, involve a community-based approach to development. This approach can lead to concerns about consistency, reliability, and security — crucial factors for any enterprise-grade application.
Opportunities Amidst Slow Adoption
However, this slow adoption phase also presented unique opportunities. Businesses that were willing to invest in understanding and customizing these models found that they offered unparalleled flexibility. Open-source LLMs allowed for more control over the data and the model itself, enabling companies to tailor the AI to their specific needs, a significant advantage over one-size-fits-all solutions.
Furthermore, the open-source community, though presenting a challenge in terms of support, also offered a wealth of collective knowledge and innovation. Companies engaging with this community found themselves at the forefront of AI advancements, contributing to and benefiting from the collective progress.
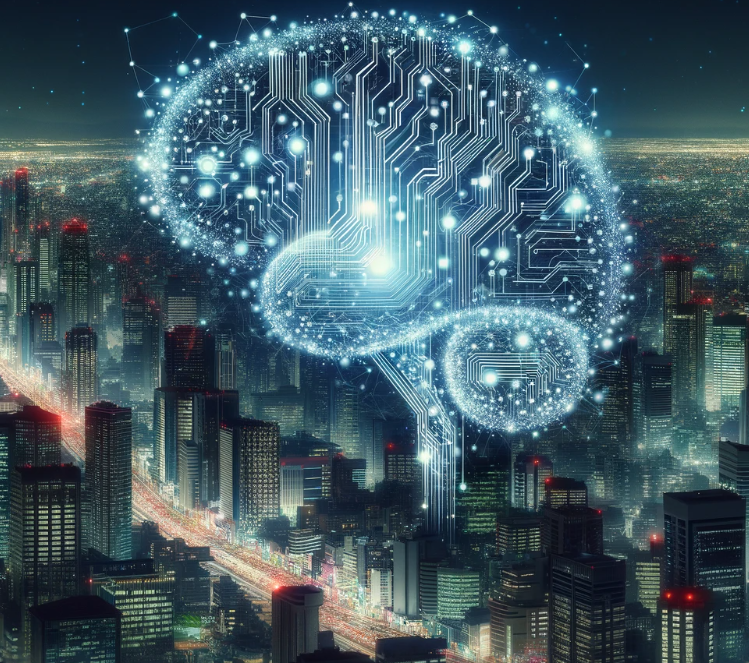
Key Players in Open Source LLMs
Few names stand out for their innovation and impact on the enterprise sector. These providers not only offer robust models but also pave the way for new applications in AI.
Meta and Llama Model
Meta, a titan in the tech industry, has been a frontrunner with its Llama model. Released in early 2023, Llama represented a significant leap in open-source AI. It was Meta’s response to the growing demand for transparent, customizable AI models in the enterprise world. Unlike closed models, Llama offers businesses the flexibility to adapt and modify the AI to fit their specific needs, a feature particularly appealing for companies dealing with unique datasets or requiring specialized AI functions.
Mistral AI and the Mixtral Revolution
Mistral AI, although a newer player in the field, has quickly risen to prominence with its Mixtral model. Launched in late 2023, Mixtral has been celebrated for its performance in various benchmarks, outshining competitors in certain aspects. Mixtral’s architecture allows for easier integration into existing systems, a key factor for enterprises looking to adopt AI without overhauling their entire tech infrastructure.
IBM, Hugging Face, Dell, and Others: Diversifying the Landscape
Other significant contributors include IBM, Hugging Face, Dell, Databricks, AWS, and Microsoft. Each of these entities brings something unique to the table:
- IBM: Known for its legacy in technology, IBM offers open-source LLMs that integrate seamlessly with its vast array of enterprise solutions.
- Hugging Face: This company stands out for its community-driven approach, making AI more accessible and collaborative.
- Dell and Databricks: These companies focus on the infrastructure aspect, ensuring that enterprises can deploy LLMs efficiently and at scale.
- AWS and Microsoft: As cloud service giants, they play a crucial role in making open-source LLMs available to a broader audience, offering robust cloud platforms for deployment.
Emerging Enterprise Open Source LLMs Use Cases
Customer Service Enhancement
One of the most prominent applications of open-source LLMs is in revolutionizing customer service. Companies are leveraging these models to power sophisticated chatbots and virtual assistants. These AI-driven systems can understand and respond to customer inquiries with remarkable accuracy and nuance, often indistinguishable from human interaction. For instance, a retail giant might use an open-source LLM to manage customer queries, providing quick, personalized responses that improve customer satisfaction and reduce the workload on human staff.
Content Creation and Management
Another significant use case is in the realm of content creation and management. Open-source LLMs like Llama and Mixtral are being employed by marketing departments to generate creative content, from product descriptions to marketing copy. These models can adapt to the company’s tone of voice and style guidelines, ensuring brand consistency across all communications. Additionally, their ability to process and summarize large volumes of information makes them invaluable for content curation and management tasks.
Data Analysis and Insight Generation
In the field of data analytics, open-source LLMs are making strides by offering deep insights from complex data sets. Enterprises are using these models to analyze customer feedback, market trends, and operational data, extracting actionable insights that can drive strategic decisions. For example, a financial services firm might use an LLM to sift through market reports and financial statements, quickly identifying key trends and investment opportunities.
Code Generation and Software Development
A more technical application is seen in software development, where open-source LLMs are being used for code generation and review. These models can assist developers by suggesting code improvements, detecting bugs, and even writing code snippets. This not only speeds up the development process but also enhances code quality. A software development company, for instance, could integrate an LLM into its development pipeline to aid in rapid prototyping and debugging.
Customized Solutions for Niche Markets
A healthcare provider could use an LLM to interpret medical records and provide preliminary diagnoses, or a legal firm might employ one to analyze case law and assist in legal research. These customized applications demonstrate the flexibility and adaptability of open-source LLMs in catering to specific industry requirements.
Advantages and Limitations of Open Source LLM
Advantages of Open Source LLMs
- Customizability and Flexibility: One of the most significant advantages of open-source large language models (LLMs) is their high degree of customizability. Unlike closed models, open-source LLMs allow businesses to tailor the AI to their specific needs, adapting to unique datasets and use cases. This flexibility is crucial for companies with niche requirements that generic models can’t fulfill.
- Data Privacy and Security: Open-source models offer enhanced data privacy, a critical concern for many enterprises. Businesses can host these models on their own servers, ensuring that sensitive information remains within their control. This aspect is particularly appealing to sectors like finance and healthcare, where data confidentiality is paramount.
- Cost Effectiveness: In the long run, open-source LLMs can be more cost-effective, especially for organizations with the infrastructure to support them. Eliminating licensing fees and reducing dependencies on external providers can lead to significant savings.
Limitations of Open Source LLMs
- Resource Intensity: Deploying and maintaining an open-source LLM requires substantial technical expertise and infrastructure. Smaller companies might find the resource requirement prohibitive, lacking the necessary computational power or technical staff.
- Inconsistent Support and Documentation: Open-source projects can suffer from inconsistent support and documentation. Unlike commercial products backed by dedicated teams, open-source LLMs rely on community support, which can vary in responsiveness and expertise.
- Language and Feature Limitations: While closed models like GPT-4 offer extensive language coverage and continually updated features, some open-source LLMs lag in these areas. This limitation can be a significant drawback for businesses requiring support for less common languages or advanced AI capabilities.
Ten Specific Enterprise Examples of Open Source LLMs
- IBM’s Extensive Utilization: IBM stands out for its broad application of open-source LLMs across various segments. From internal tools like their HR app utilizing their own Granite model, to advanced consulting solutions leveraging Llama 2, IBM exemplifies the multifaceted potential of open-source LLMs in large enterprises.
- Wells Fargo’s Strategic Internal Use: The adoption of Meta’s Llama 2 model by banking giant Wells Fargo for internal applications underscores the growing trust in open-source LLMs within the highly regulated financial sector.
- Shopify’s AI-Powered Sidekick: Shopify’s implementation of Llama 2 in their Sidekick tool illustrates the transformative impact of open-source LLMs in e-commerce. Sidekick aids small business owners with tasks like generating product descriptions, epitomizing practical AI utility in online commerce.
- VMWare’s Adoption of HuggingFace’s StarCoder: VMWare’s integration of StarCoder for developer efficiency demonstrates the strategic alignment of open-source LLMs with core business processes, highlighting their relevance in technology sectors.
- Walmart’s Conversational AI Applications: Retail titan Walmart’s use of various AI applications, including a mix of GPT-4 and open-source models for customer care chatbots, reflects a comprehensive and pragmatic AI strategy in the retail industry.
- Intuit’s GenOS Integration: Intuit’s use of open-source LLMs in their Intuit Assist feature is a testament to the adaptability of these models in specialized corporate environments, particularly for tasks like customer support and analytics.
- Brave’s Leap to Mixtral 8x7B: The security-focused browser Brave’s shift to Mistral AI’s Mixtral 8x7B for its assistant Leo highlights the importance of privacy and independence in technology choices, setting a precedent in the tech industry.
- Gab Wireless’s Child Safety Measures: Gab Wireless’s implementation of Hugging Face’s open-source LLMs for child safety showcases how AI can be utilized for societal benefits, particularly in ensuring secure digital experiences for younger users.
- LyRise’s Innovative Recruitment Bot: The use of Llama in LyRise’s recruitment chatbot, which helps businesses find AI and data talent in Africa, exemplifies the global reach and diverse application potential of open-source LLMs.
- Niantic’s Enhancement with Peridot: Niantic’s integration of Llama 2 in Peridot for generating interactive, environment-specific reactions in gaming illustrates the creative and experiential dimensions open-source LLMs can bring to the entertainment sector.
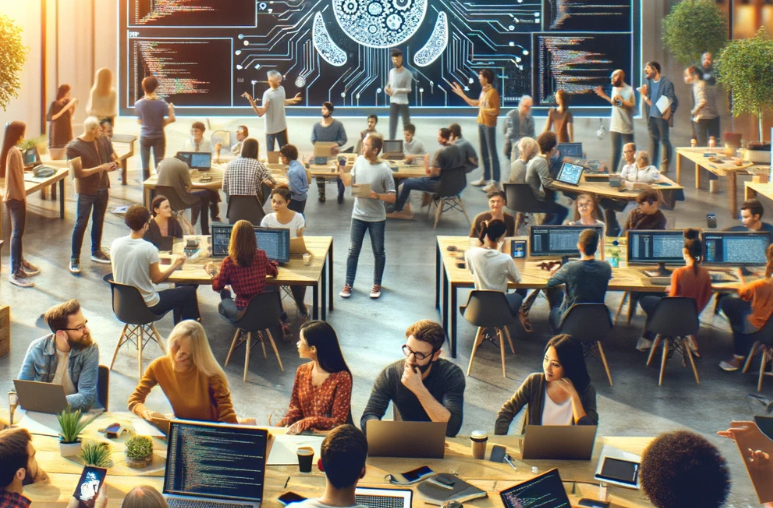
The Future of Open Source LLMs in the Enterprise
This evolution is not merely about the technology itself but how it reshapes the interaction between AI and business processes, fostering a new era of innovation and efficiency.
A. Increasing Customization and Specialization
One of the most compelling prospects for open-source LLMs lies in their inherent flexibility. Businesses are increasingly seeking to tailor AI solutions to their specific needs. Open-source models offer a unique advantage in this regard, allowing enterprises to customize and fine-tune the models to suit their unique data sets and operational requirements. This level of customization is critical in industries where specificity and accuracy are paramount, such as in legal, medical, and technical fields.
B. Enhanced Collaboration and Community Development
The open-source nature of these LLMs encourages a collaborative approach to AI development. As more enterprises adopt and contribute to these models, we can expect a rapid acceleration in their capabilities and applications. This collaborative environment not only speeds up innovation but also democratizes AI development, allowing smaller players to contribute and benefit from the collective progress.
C. Balancing Cost and Control
Cost-efficiency remains a crucial factor for businesses. Open-source models provide a cost-effective alternative to proprietary models, especially for companies with the infrastructure to support them. Moreover, they offer enterprises control over their data and models, a critical consideration in an era increasingly concerned with data privacy and security.
D. The Blending of Open and Closed Models
Looking ahead, we’re likely to see a more nuanced approach to LLM deployment in enterprises. Instead of a binary choice between open and closed models, businesses will increasingly leverage a hybrid model, combining the strengths of both. This approach will enable enterprises to use open-source models for tasks requiring high customization and control, while still benefiting from the advanced capabilities and ease of use provided by closed models.
E. Preparing for Regulatory Changes
As LLMs become more integral to business operations, regulatory scrutiny is inevitable. Open-source models offer the flexibility to adapt quickly to regulatory changes. Businesses using these models can modify them to comply with new regulations, ensuring a smoother transition and continued compliance.
TL;DR
- Trend: Shift from proprietary to open-source LLMs for flexibility and cost savings.
- Advantages: Customization, transparency, and community involvement.
- Challenges: Support concerns, resource intensity.
- Key Players: Meta, Mistral AI, IBM, Hugging Face, etc.
- Use Cases: Chatbots, content creation, data analysis, niche solutions.
- Future: More customization, hybrid models, regulatory adaptability.