AI & Design: takeaways from SXSW
Insights from the technology & arts conference of the year
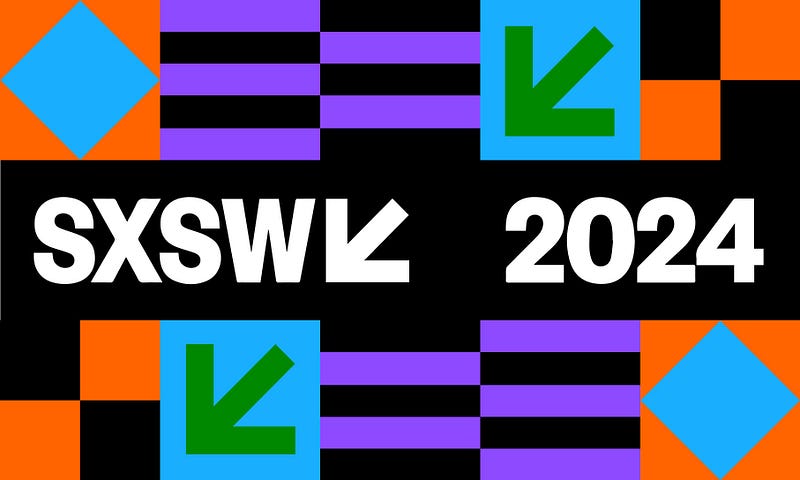
SXSW just wrapped last week. Here’s everything you need to know about design and AI from the arts & technology conference of the year. From addressing concerns about AI, to the AI-innovation gap, industry insights, challenges & future visions.
(1) Design Against AI, John Maeda
(2) AI + UX: Product Design for Intelligent Experiences, Ioana Teleanu
(3) Designing Successful AI Products and Services, John Zimmerman, Nur Yildirim
(4) Creativity in Flux: Art & Artificial Intelligence, Brooke Hopper, Debbie Millman
(5) Designing for AI, Marco Barbosa, Hjörtur Hilmarsson
(6) The Great Interface Shift: Five Trends to Know in 2024, Jake Brody
1. Design Against AI, John Maeda
Evolving dynamics between design and AI, whether to compete with, protest against, or collaborate with AI, & the need for continuous learning
3 points: (1) understand computation to get beyond the myth of AI, (2) work will transform faster for some than others, (3) Design’s role to make things desirable, valuable, ethically-considered is a challenge ahead
Brief history. LLMs, conversational interfaces, chatbots… aren’t new. Nicholas Negroponte envisioned a conversational prediction model in 1967. Erika Hall’s Conversational Design emphasizes voice is the oldest interface because it feels most natural. MIT’s Dr. Weizenbaum, inventor of the first chatbot, warned in 1966:“short exposures to a relatively simple computer program could induce powerful delusional thinking”
People have been working on AI for decades. LLMs grew exponentially from 2014 to 2022. Generative AI exists because of the transformer. Learn its comprehensive history from BERT to ChatGPT.

What are LLMs?
The ‘simple physics’ definition: Imagine the LLM as a genius locked in a room. It can receive only one note under the door & use the note only once (token window limit). It can use only what’s inside the room and on your paper, then confidently take a guess (no context). After returning an answer, the genius forgets everything you wrote to them (stateless).
LLMs get it wrong because people get it wrong. People and AI are both bad at predicting the future, good at knowing what happens next if it happened before, Rumsfeld Matrix.
Other ways to understand AI & LLMs:
- Ted Chiang’s ChatGPT is a blurry JPEG of the web. It’s nice to have a blurry JPEG, but let’s also stick with high fidelity human reality
- Josh Clark’s AI as your new design material, just like pen and paper, but with indeterministic outputs
- John Oliver’s Artificial Intelligence: Last Week Tonight is well-informed, educational, fun
Robots, Spacial Computing
Robots are getting smarter, stronger, faster. Tesla Optimus can fold laundry. Figure Bot 1 learned to make coffee by watching people make coffee. Boston Dynamics Atlas can pick up heavy things. Unitree H1 can run 3.3 m/s, the current world record.
Apple Vision Pro. Spacial Computing is back, here’s what leaders need to know. Ivan Sutherland developed the first advanced VR system in the 1960s. Learn more from the book on everything spacial computing AR/VR.
ABC’s of AI
A. Large models, small models. Large models do in-depth reasoning. Small models run faster, on the edge. But distinctions are blurring.
B. Open models, closed models. LLaMA can be modified by anyone. OpenAI models are protected and can not be modified.
C. Mono-modal, multi-modal. Models with one modality are specific & focused. Combining modalities like image + sound, video + language… will increase over time and benefit from diverse perspectives.
Terminology keeps growing: embeddings vs. completion models, full model fine-tuning vs. LoRA fine-tuning, function calling models, agents etc. Agents will be big in the user experience category, useful for many things beyond prompting.
Categories of craft: Handcrafted vs. Speed-crafted
Handcrafted things feel authentic and delights the maker in creating them. See Rivian’s Lock Chirp, Dieter Rams inspired design components, typography morphing itself based on semantics.
Speed-crafted things are made fast, and getting faster with AI. Images generated quickly, playHT text-to-speech, Yokhei Nakajima’s PredictiveChat with negative latency that can guess what you’ll say before you say it.
Makers’ lives are changing
Designers’ processes and tools are changing. Engineers’ processes and tools are changing. Product Managers are experiencing capability enhancements, collapsing the product stack.
Marketing and product loops are being impacted. Marketing is the buyer’s experience, product is the customer’s experience.
- Marketing design tools: copy creation, image creation, podcast creation, video editing, presentation creation
- Product design tools: customer support, software development, product development, zero UI generated on the fly with a function-calling model, teleporting people to their goal without a journey.
If you don’t like change, you’re going to like irrelevance even less (Eric Shinseki)
Critical thinking & critical making
Both take time. Both are needed for AI. For-profits are not good at critical thinking, making useful things with unintended consequences. Non-profits are good at critical thinking, acting as well-informed critics not caught in cycles of executing products. See standards and tools for critical thinking.
Being human is TBD
Technology challenges us to assert our human values which means that first of all, we need to figure out what they are (Sherry Turkle)
There are tasks humans can do, tasks AI can automate, and a new category of tasks humans can do only with the help of AI:
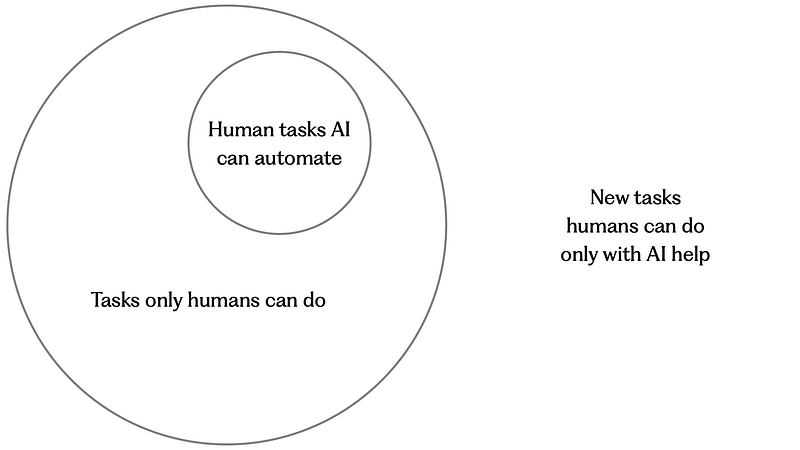
Towards the future: Design humanizes technology, but we often hear “don’t humanize AI.” Design’s challenge is to figure out net-new AI value. While doing so, remember AI is not a person, it’s math.
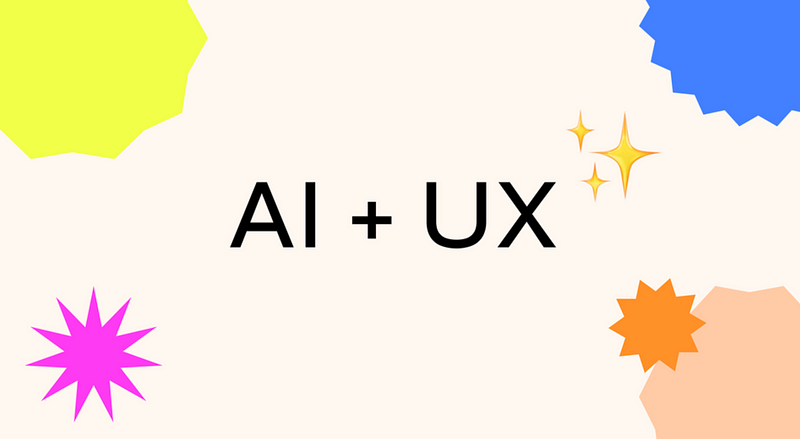
2. AI + UX: Product Design for Intelligent Experiences, Ioana Teleanu
Getting to know AI’s challenges & opportunities, what AI can and can’t do
Use AI
More people using AI tools will help expose limits, boundaries, understand where products fail, what they should & shouldn’t do. Although AI has moderate performance for most tasks, it’s here to stay.
Gartner’s Hype Cycle suggests we are coming down from the ‘peak of inflated expectations’ for generative AI. Many companies are retrofitting AI into their products in a scramble to remain competitive. Use cases are unclear. Customers tend not to trust things they don’t understand.
What is AI?
(1) From Elements of AI, AI is any system with autonomy and adaptivity, meaning it can perform complex tasks without user guidance and improve & learn based on usage
(2) From Andrew Ng, AI is a collection of tools, and tools are the methods by which we teach AI systems to do things. We shape our tools, and thereafter, our tools shape us, John Culkin
AI Challenges & Opportunities
- Bias in AI systems reflect bias in human society data. Opportunity to decide what datasets should be part of our future. Many companies already doing this. Curate what to perpetuate with data.
- Safety & security. Any emergent tech can be exploited with bad actors. We don’t have the right systems to minimize harms yet. To design AI systems that feel safe, anticipate all the ways they are not.
- Design for probability. Conventional interactions have predictable behavior. For example, if this happens, present users with option A; if that happens, option B. But AI systems output things that are unpredictable. You don’t know if results will be good, meeting user expectations. Feedback mechanisms are one solve.
- Trust & transparency. People struggle to trust things they don’t understand. AI hallucinates outputs. Consider explainable AI concepts, showing people how systems arrived at the result.
- Ownership and IP. Prediction that society will change what we value. Things may be valuable if created by a human, triggering emotional responses. Less value may be placed on things easy to produce.
- The future is hybrid, but start with people. Humans will have AI partners. When everyone has an AI partner, make sure to perpetually put users in the driver’s seat.
- Fight bias. Give people instruments to give feedback to AI systems, report undesirable situations, recover from mistakes.
- Build for everyone. Build accessible AI without widening privilege & knowledge gaps.
- Fail gracefully, promote good. Make products that are ethically-considered. For example, the ‘like’ button gives positive feedback but has harmed teenagers’ mental health.
- Help people grow. Humans and AI learn and teach each other, see mutual learning.
- Age nicely. Tech changes, so build things with a level of abstraction that will evolve with the future in mind.
Interaction Design
AI is a new UI paradigm. See Jakob Nielson’s intent-based outcome specification where people specify goals for AI systems to accomplish.
From web to mobile, people stuffed things into smaller screens. Instead of retrofitting AI, consider how to design AI-native products, intentionally embedded into user experience. 2 examples: (1) auto-generated chapters from Loom, (2) browser website quick preview without having to open tabs to see content.
AI can improve UX in 5 ways
- Relevance. Hyper-personalization to your preferences, context-awareness, meeting you where you are, appearing in the right time.
- Decluttered. There’s an app for everything right now, but the future holds experiences built on intent. Consider products with one interface, like Rabbit R1 exemplifying vision for future AI hardware.
- Accessibility. Opportunity to leverage AI to improve accessibility. Current examples include alt text, screen readers & image recognition for the visually impaired. Lots of white space to explore.
- Helpful. Answer questions in specific & relevant way, bespoke to you
- Improve learning. AI can facilitate user testing of design concepts quickly, accelerate learning that informs design decisions with AI
Human vs. AI?
AI can’t replace people because (1) AI systems can’t figure out the right problems to solve, (2) AI systems can’t understand complex social landscapes around people
Incorporate AI into Design Process
AI tools help understand, augment thinking, cover blind spots in design processes. AI can help with brainstorming, accelerating idea to execution. Designers may become curators rather than generators of design. One example is UIzard’s autodesigner.
Competitive advantage comes from using AI tools and discovering the right human problems to solve with AI. Think critically about whether we should build something, with humans & ethics at the center.
Remember, AI is a tool. You (makers) have agency.
3. Designing Successful AI Products and Services, John Zimmerman, Nur Yildirim
AI challenges & opportunities, based on 10 years of research. A talk from my advising professor and a friend from Carnegie Mellon.
Historically, most AI products have been designed by people with a P.h.D in Machine Learning. This talk addresses the AI Innovation Gap, why products fail, how to actually innovate with AI in 5 key takeaways.
What is AI?
Everything from spam filters to systems that diagnose cancer, from maps re-routing to drug discovery. What remains consistent across all AI applications, is the idea of uncertainty. You never know if the system you are trying to create can be built, or the errors it can make.
More examples: Kiva Robots run around, go under shelves, lift shelves, organize warehouse inventory. Unlike humans, they’re spread out & trained on reinforcement learning. Airbnb smart pricing analyzes the maximum you can charge for space and still have it likely rent, giving CEO power to mom and pops.
LLMs, ChatGPT, foundation models… has created a lot of opportunity. Its tremendous success implies we have a great innovation process. Research shows this is not true.
AI Innovation Gap
Today, 85% AI projects fail before deployment. They also fail after deployment because
- AI systems can’t achieve good enough performance and are not useful
- AI systems don’t generate enough service value. For example, Alexa did not result in people ordering more products from Amazon. Instead, every time people ask Alexa to play music, Amazon loses money.
- AI systems don’t solve a human problem. For example, healthcare clinical decision systems offer predictions, but doctors don’t need predictions during common scenarios. Instead, doctors want assistance during uncommon scenarios, which AI is not good at
- Ethics, bias, privacy, societal harm. For example, systems can judge how long someone should go to jail by predicting likelihood they will commit future crime. But systems are inherently racist, since they reflect human biases in the data.
- People miss low-hanging fruit. There are simple AI opportunities aligned with user and service goals, but companies don’t build them. For example, Instagram wants influencers to post to attract eyeballs, but never learns which tags influencers frequently use, forcing influencers type the same tags repeatedly. Not hard, but an unnoticed AI opportunity. Self-parking cars serve only a tiny group of users who live in suburbs and are afraid to park in the city. It starts with the prediction: Is this space big enough? This simple feature could be on every car, but there’s a mindset we need to make AI capabilities that are hard, as opposed to things that are useful.
More about practical AI challenges:
Invention vs. Innovation. Invention is creating new capabilities. Designers’ secret sauce is innovation, remixing and giving new form to existing things in new ways. Recognizing human needs, then giving it a form that people find valuable.
Designers tend to have a poor understanding of AI and think of things that can’t be built. Data Scientists are far removed for users and think of things that people don’t want.
Why AI Products Fail
High failure rate of AI projects can be attributed to the following reasons:
- Teams are choosing the wrong thing to make. The Data Science process starts with quick problem formation, then move on. They’re not trained to ideate, to think of hundreds of things to do that are high value & low risk. Teams tend to pick something then build, & not ask for all the different things we can do towards accomplishing the goal, before building it. Healthcare is a great example. AI does well for textbook cases. But doctors are really looking for help regarding edge cases.
- Designers are typically not invited to particpate until the decision of what to build has already been made. Designers also tend to think of things AI actually cannot do. Can also blame the media, where AI is hyped as superhuman.
- Designers have internalized capabilities, abstractions to draw from when crafting experiences. However, AI capabilities feel distant because AI literature is all about mechanisms, how it works. Think support vector machines, neural networks, etc. Almost none of it describing capabilities, what AI can do.
- AI-led problem framing. If you’ve already decided the solution is AI, you’re not really doing user-centered design. Think classic double diamond. With AI, you’re starting in the middle of the double diamond. User-centered design falls short. Technology-centered design also doesn’t work.
- Hard to estimate cost of building & running a system. If you go to an architect, they can tell you what you can get with $100M. If you go to a data scientist with $100M, they struggle to estimate.
Ideation is a valuable technique to shift dialogue from should we or shouldn’t we do this one option, into considering all other options that are equally valuable & lower risk from a responsible AI POV
Things people have tried:
- Accenture Song observed Designers and Data Scientists working together closely at the beginning of a project. Adding a ‘data’ swim line into the experience design pipeline helped.
- Annotating UX wireframes with data sources adds more accountability on where data comes from.
- From examples where AI is commercially successful, 8 high level AI capabilities emerged. However, giving Designers these capabilities to ideate from were not successful. Giving Designers many examples of AI first, then presenting situations where AI can succeed with speed and scale but not a lot of human expertise, was successful. Methods documented here.
5 ways to Innovate AI products & Services
- Don’t replace an expert. Instead, look for situations where you want an unlimited number of interns. Even better, you wouldn’t even hire an intern because the task is so tedious. Look for places where something is better than nothing. Remember AI can do an OK job, not a great job.
- Think about where you are in the innovation process. Versioning, Visioning, Venturing. UX can add to versioning, thinking from the user perspective for incremental innovations. Service design can add to visioning, thinking strategically to find low-risk, high-value opportunities.
- Design can facilitate the conversation, bring diverse perspectives together, ideate all the different ways to achieve a goal.
- Ideation, & scaffolding others to ideate. Design can hold the team accountable where looking for situations moderate performance can have significant value.
- Look for opportunities for moderate AI performance. When you find ideas where moderate performance works, you almost always avoid responsible AI issues. Paper on this coming soon.
4. Creativity in Flux: Art & Artificial Intelligence, Brooke Hopper, Debbie Millman
Evolution of creative software, potential of generative AI, role of humanity
Interview with Adobe AI/ML Designer. The dialogue takes a creative angle, focusing on empowering artists, writers, makers.
Difference between AI, ML, generative AI? Generative AI is a subset of Deep Learning, which is a subset of ML, which is a subset of AI. AI has been used for decades across all sectors: banking fraud, spellcheck, photoshop’s content-aware fill, etc.
AI impacted by human bias? Yes, data comes from humans and humans have bias. For example, systems to approve people for loans are racist. How might we design & curate data for desirable futures?
Protecting artists and creators’ IP? Transparency in how AI makes decisions? Methods include embedding data into content itself, regarding how and when it was created. Also tagging content with do-not-train credentials. For example, Adobe has the option to turn on ‘content credentials’ to track everything you do embedded in the .psd. ‘Image abuse’ is when your images are used without your permission. There’s still white space to ensure the right guardrails, rules, regulations…
What if people can decide when they’re contributing their own data to train models? Still grappling with this, but (1) transparency needs to come from companies, and (2) education can and should be provided to people who sign in to sites that opt-in your data. Becoming more informed on website security, spotting AI content and deepfakes is important.
Becoming dependent on AI to crafting things? Humans and machines are different. Humans have unique qualities: emotion, experience, rule-breaking based on circumstance, questioning why and deciding what are the right things to build. Machines struggle to replicate these qualities.
How can educators ensure students don’t use AI to plagiarize? Less about not using AI, more about adapting. One example is instructors giving students papers written by AI, for students to make corrections. Universities are putting in AI guidelines.
Examples of artists using AI in compelling ways? Pum Lefabur, from Design Army created a whole ad campaign from AI. Marian Bantjes also crafting art with AI. Upcoming MoMA exhibit where a Furniture Designer using generative AI to re-imagine what iconic furniture would look like if they were crafted by Designers of color.
AI and imagination? AI sits alongside imagination, pushing imagination. AI serves weird results sometimes. For example, Adobe Firefly wanted to put human faces on any living thing, like a chihuahua with human face. But this may spark interesting new directions.
[Speculation] AI-enhanced imagination is original? Does AI enhance ideas or fuel variations? Fuzzy bounds. Hard to determine this, but all comes down to intent. AI image editors are improving, enabled by mechanisms like control-net to illustrate depth of images.
Designing with AI tools? With AI tools, more people will be able to access more mediums and get started faster. You can give a Designer and non-Designer the same set of tools. The Designer’s output will still likely be better in the end, due to having aesthetic sensibility.
What’s unique about Generative AI? The same result can’t be generated twice, due to its ‘generative’ nature.
[Speculation] What’s in store for the future? Art will be embedded in society. We’ll see more creativity, immersive experiences. Art won’t be something you go see. We’ll become participants. Art will come to us and become part of who we are.
5. Designing for AI, Marco Barbosa, Hjörtur Hilmarsson
Design can make AI desirable and useful. 3 stories from developing 3 different AI experiences: conversational AI, co-pilots, immersive experiences.
1. Conversational AI
14Islands built Aila, a conversational agent for their website.
Learnings. Information transparency makes AI systems trustworthy. People trust systems more when they know where information is sourced, can evaluate the credibility of an answer, and know when systems are hallucinating and providing inaccurate responses. It’s important to test with typical FAQ questions. Add guardrails for sensitive data & questions you don’t want your agent to get into (e.g. openAI’s moderation API to flag questions), and provide standard answers. Design the personality of your agent. Include follow-up prompts to keep the conversation going and help people get started. Keep improving the system.
Design approach. A self-evaluation system was built within their conversational agent. Credible answers are labeled as confident. Futuristic & creative answers are labeled as hallucination. For hallucinated answers, background changes from deep blue to vibrant colors.
2. Co-pilots
AI as a partner can enhance experience, improve efficiency and productivity, while keeping people still in control.
Great opportunity to provide highly contextual experiences, serving results you didn’t know you need, in the right moment.
Case study: Seekout’s automated recruiting platform faced a challenge: How to craft useful descriptions for each company’s context? The same job description needs to be very different for different companies. This is a highly repetitive, cumbersome task.
Approach: Opportunity mapping was utilized to come up with list of things people want, then map to things that could be achieved with AI. It resulted in Assist, a feature that will help speed up the search for candidates. When in use, their logo turns into an asterisk to indicate that AI is happening. Two capabilities were built: (1) AI systems sifting through large quantities of data, then generating a quick summary people can turn on or off, and (2) AI systems generating interview questions based on the employer’s job description and candidate’s resume, saving time for both stakeholders.
3. Immersive Experiences
Speculative view of the future of AI with emergent tech capabilities, particularly multi-modal experiences and spacial computing.
Insights embedded in life. Imagine UI appearing on the fly in extended reality. Experiencing immersive real-time collaboration, communication. See Pluto, a virtual reality chat app to talk to someone else, fooling the brain into thinking you are physically in the same space.
Branding for spacial computing. Consider branding that exists in a 3D space. People can touch the brand. You’ll need to think about materials, how people sense the environment, motion & sound.
Real-time accessibility. This is where design can bring magic. In spacial environments, you are not tied to the laws of physics, and can leverage human senses.
Create magical moments. Embrace the opportunity for creative expression with AI. Try opportunity mapping to discover out of many ideas, where AI can be applied. Use motion to communicate change, & guide people toward desired results. Maintain clarity and information transparency to continuously build trust with users.
6. The Great Interface Shift: Five Trends to Know in 2024, Jake Brody
5 trends exploring the decline of customer obsession, the influence of generative AI, the stagnation of creativity, the balance of tech benefits and burden, people’s new life goals. See Accenture Life Trends 2024.
Where’s the love?
Decline of customer obsession. Customers experienced whiplash on profit-gaining maneuvers, like shrinkflation (same price, reduce quantity) and skimpflation (same price, reduce quality of service). People are skeptical of purpose-driven marketing, whether for the benefit of customers or the business. But when customers are wary of one brand, they become wary of others. Exception is Costco, seeing 15% growth in business by keeping prices consistent when other brands raised prices. Another is Apple, with strong brand loyalty and customers already bought into the ecosystem.
The Interface Shift
Information discovery from search to conversation, from “I want..” to “I want to..” This interaction enables different relationship types, with different levels of intimacy between humans and machines.
Flexible personalization & relevance redefines commerce. Customers can tell brands what they want with context, interacting with brands through AI moderated dialogue. Multi-modal inputs encourage people to learn to mix text, image, sound with context, removing barriers with instant translation, & keeping people engaged with hyper-personalization.
Customers will also use technology against brands. AI may find better suited products for people, but from competitive brands. This is a challenge for companies to strengthen brands, regain customer loyalty, retrain customer experience staff to handle interaction with AI, or train AI agents to be bought into the brand.
Mediocrity & Creative stagnation
Caution against technology becoming the tastemaker, rather than people. One example is algorithms controlling content we see and preventing from seeing new things.
There are concerns that originality & creativity is being outcompete by things created quickly & efficiently. Efficiency culture exacerbates this, with risk toward leaning on reliability in ways of the past, over bold originality. Stand out by doing what the majority is not.
Design by data. Data is powerful, but data tends to point toward familiarity. Brands fall into the trap of similarity. Hard for creative differentiation. Therefore, focus on what matters, including customer loyalty.
Balance of Benefits & Burden
There’s growing unease that technology is something that happens to people, rather than something that happens for people.
Lack understanding of technology changes makes the future feel daunting. Society should have a voice toward risk, demand regulatory action, become part of the solution.
People’s new life goals
There are pressing need for brands to embrace the changing relationships. Organizations need to be thoughtful regarding how technology fits into life. People reconsider what they do and how to engage based on what new tools. Mindsets redefine what people want and need from brands. Brands should learn what new mindsets of how people live and what they want. Having a life-centric approach enables brands to think about consumer.
Thanks for reading. More from the design track.